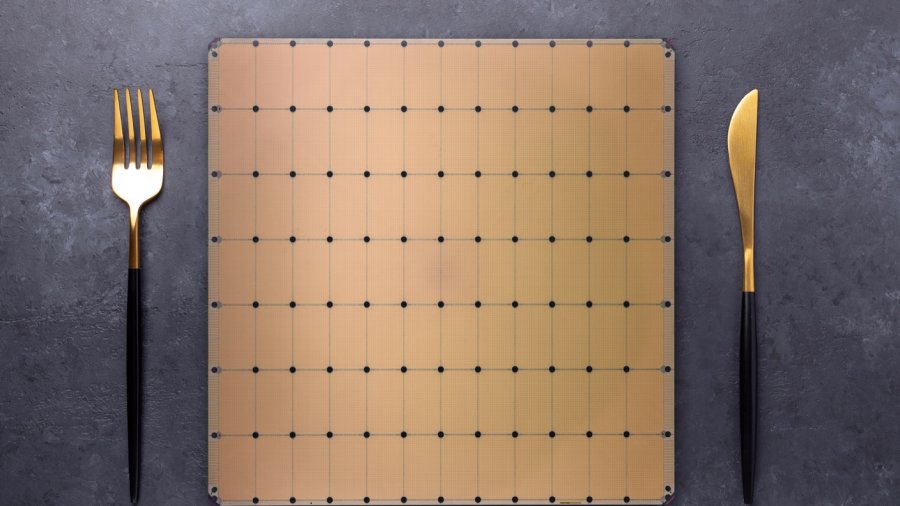
The world’s biggest AI chip just doubled its specs—without adding an inch.
The Cerebras Systems Wafer Scale Engine is about the size of a big dinner plate. All that surface area enables a lot more of everything, from processors to memory. The first WSE chip, released in 2019, had an incredible 1.2 trillion transistors and 400,000 processing cores. Its successor doubles everything, except its physical size.
The WSE-2 crams in 2.6 trillion transistors and 850,000 cores on the same dinner plate. Its on-chip memory has increased from 18 gigabytes to 40 gigabytes, and the rate it shuttles information to and from said memory has gone from 9 petabytes per second to 20 petabytes per second.
It’s a beast any way you slice it.
The WSE-2 is manufactured by Taiwan Semiconductor Manufacturing Company (TSMC), and it was a jump from TSMC’s 16-nanometer chipmaking process to its 7-nanometer process—skipping the 10-nanometer node—that enabled most of the WSE-2’s gains.
This required changes to the physical design of the chip, but Cerebras says they also made improvements to each core above and beyond what was needed to make the new process work. The updated mega-chip should be a lot faster and more efficient.
Why Make Giant Computer Chips?
While graphics processing units (GPUs) still reign supreme in artificial intelligence, they weren’t made for AI in particular. Rather, GPUs were first developed and used for graphics-heavy applications like gaming.
They’ve done amazing things for AI and supercomputing, but in the last several years, specialized chips made for AI are on the up and up.
Cerebras is one of the contenders, alongside other up-and-comers like Graphcore and SambaNova and more familiar names like Intel and NVIDIA.
The company likes to compare the WSE-2 to a top AI processor (NVIDIA’s A100) to underscore just how different it is from the competition. The A100 has two percent the number of transistors (54.2 billion) occupying a little under two percent the surface area. It’s much smaller, but the A100’s might is more fully realized when hundreds or thousands of chips are linked together in a larger system.
In contrast, the WSE-2 reduces the cost and complexity of linking all those chips together by jamming as much processing and memory as possible onto a single wafer of silicon. At the same time, removing the need to move data between lots of chips spread out on multiple server racks dramatically increases speed and efficiency.
The chip’s design gives its small, speedy cores their own dedicated memory and facilitates quick communication between cores. And Cerebras’s compiling software works with machine learning models using standard frameworks—like PyTorch and TensorFlow—to make distributing tasks among the chip’s cores fairly painless.
The approach is called wafer-scale computing because the chip is the size of a standard silicon wafer from which many chips are normally cut. Wafer-scale computing has been on the radar for years, but Cerebras is the first to make a commercially viable chip.
The chip comes packaged in a computer system called the CS-2. The system includes cooling and power supply and fits in about a third of a standard server rack.
After the startup announced the chip in 2019, it began working with a growing list of customers. Cerebras counts GlaxoSmithKline, Lawrence Livermore National Lab, and Argonne National (among others) as customers alongside unnamed partners in pharmaceuticals, biotech, manufacturing, and the military. Many applications have been in AI, but not all. Last year, the company said the National Energy Technology Laboratory (NETL) used the chip to outpace a supercomputer in a simulation of fluid dynamics.
Will Wafer-Scale Go Big?
Whether wafer-scale computing catches on remains to be seen.
Cerebras says their chip significantly speeds up machine learning tasks, and testimony from early customers—some of which claim pretty big gains—supports this. But there aren’t yet independent head-to-head comparisons. Neither Cerebras nor most other AI hardware startups, for example, took part in a recent MLperf benchmark test of AI systems. (The top systems nearly all used NVIDIA GPUs to accelerate their algorithms.)
According to IEEE Spectrum, Cerebras says they’d rather let interested buyers test the system on their own specific neural networks as opposed to selling them on a more general and potentially less applicable benchmark. This isn’t an uncommon approach AI analyst Karl Freund said, “Everybody runs their own models that they developed for their own business. That’s the only thing that matters to buyers.”
It’s also worth noting the WSE can only handle tasks small enough to fit on its chip. The company says most suitable problems it’s encountered can fit, and the WSE-2 delivers even more space. Still, the size of machine learning algorithms is growing rapidly. Which is perhaps why Cerebras is keen to note that two or even three CS-2’s can fit into a server cabinet.
Ultimately, the WSE-2 doesn’t make sense for smaller tasks in which one or a few GPUs will do the trick. At the moment the chip is being used in large, compute-heavy projects in science and research. Current applications include cancer research and drug discovery, gravity wave detection, and fusion simulation. Cerebras CEO and cofounder Andrew Feldman says it may also be made available to customers with shorter-term, less intensive needs on the cloud.
The market for the chip is niche, but Feldman told HPC Wire it’s bigger than he anticipated in 2015, and it’s still growing as new approaches to AI are continually popping up. “The market is moving unbelievably quickly,” he said.
The increasing competition between AI chips is worth watching. There may end up being several fit-to-purpose approaches or one that rises to the top.
For the moment, at least, it appears there’s some appetite for a generous helping of giant computer chips.
Image Credit: Cerebras
* This article was originally published at Singularity Hub
0 Comments