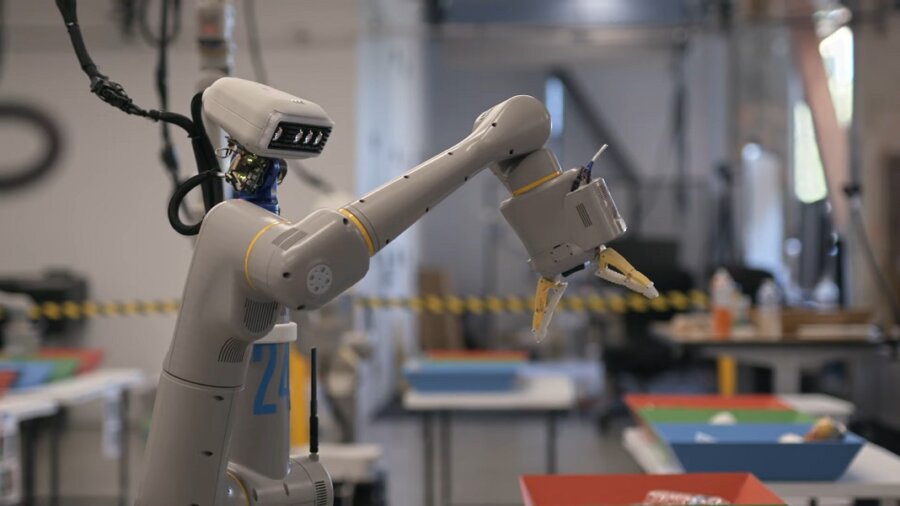
People have been dreaming of robot butlers for decades, but one of the biggest barriers has been getting machines to understand our instructions. Google has started to close the gap by marrying the latest language AI with state-of-the-art robots.
Human language is often ambiguous. How we talk about things is highly context-dependent, and it typically requires an innate understanding of how the world works to decipher what we’re talking about. So while robots can be trained to carry out actions on our behalf, conveying our intentions to them can be tricky.
If they have any ability to understand language at all, robots are typically designed to respond to short, specific instructions. More opaque directions like “I need something to wash these chips down” are likely to go over their heads, as are complicated multi-step requests like “Can you put this apple back in the fridge and fetch the chocolate?”
In contrast, a new breed of massive language models inspired by Open AI’s groundbreaking GPT-3 are capable of some impressive linguistic feats. By training on enormous amounts of written material scraped from the web, these AI systems are able to generate high-quality prose, power convincing chatbots, and answer complicated questions about text.
Google has attempted to combine the two in a new project aimed at boosting robots’ ability to understand us. By combining its PaLM large language model with robots made by Everyday Robots—a spinoff from Alphabet’s “moonshot factory,” X—they’ve built prototype mechanized butlers that can do a human’s bidding around the house.
The robots, which roll around on wheels and feature a single robotic arm and a sensor-packed head, were first trained to carry out a variety of basic actions by human operators who remotely controlled them through a series of tasks.
Engineers then created new control software that taps into PaLM’s language skills to translate spoken or written commands from a human into the actions required to achieve it. The software takes advantage of an approach called “chain of thought prompting” that Google unveiled earlier this year, which enables models to break down problems into a series of intermediate steps.
It uses this to divide requests into smaller sub-problems that it can solve with its pre-trained suite of actions. For instance, “get me a Coke” might be converted into “go to the kitchen, open the fridge, pick up a Coke, and return to the living room.”
The robots were given 101 instructions by human users and were able to come up with a sensible response 84 percent of the time, and actually pull them off seamlessly 74 percent of the time.
That represented a 14 percent and 13 percent improvement, respectively, when compared to robots using a less powerful language model than PaLM, Google’s head of robotics Vincent Vanhoucke said in a blog post. The robots powered by PaLM also saw a 26 percent boost in their ability to carry out complicated multi-step requests.
This is still very much a work in progress, though, and the robots can still be thrown off by things as simple as a change in lighting or moving objects out of their familiar positions, according to Wired. It’s not clear whether the language comprehension problem is really more pressing than actually getting robots to successfully carry out tasks in the ever-changing real world.
But the researchers hope the benefits could run in the other direction too, by giving large language models a way to interact with the physical world. While it isn’t yet clear how this project could be used to actually retrain these models, it could be one way to start grounding AI’s language skills in the real world.
So whether or not this line of research ever leads to robotic butlers becoming a reality, it seems likely to push the fields of both robotics and AI towards new and powerful capabilities.
Image Credit: Everyday Robots
* This article was originally published at Singularity Hub
0 Comments